Globally, over 90 percent of agents leave their organization to pursue opportunities outside the insurance industry. Thus making the agents onboarding process a continual recurrence for the insurance companies.
Organizations, as well as agents, crave for easy onboarding instead of long trails of paperwork. Simplifying documentation and data entry right from the beginning can be a great win for organizations looking to improve operational efficiencies. And it is, in fact, possible to automate insurance agents onboarding processes.
Problems In Onboarding Insurance Agents
#Finding the Right Person
One of the major problems that insurance companies face is the agent’s intent towards making profits. There sure is the pressure of earning commissions forcing agents to sell policies that bring greater profits to them.
Insurance companies indeed need to find agents aligned with organizations’ ethics and not driven by personal benefits.
#Operational Cost in Recruitment
When an organization issues a circular for hiring agents, thousands of applications flood in. The human effort in shortlisting candidates is time-consuming and can increase operational costs by 30%-60%.
Automating ‘Traditional Onboarding’ Processes
Organizations can introduce an “apply online” portal where prospective agents can upload documents directly required for employment qualifications. Post this, the shortlisting of candidates can be automated, which is otherwise done manually even today.
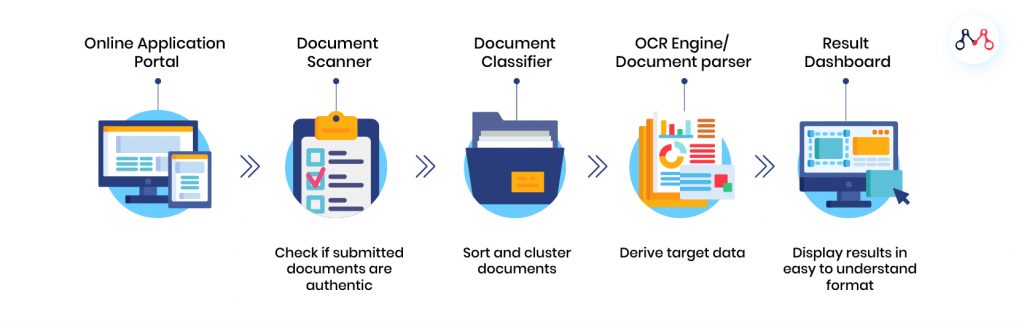
#Check Authenticity of the Submitted Documents
Smart document scanners identify the authentication rules (holograms, unique id, etc.) and accordingly process it for the next steps.
#Sort and Cluster Documents
Document Classifiers can cluster documents based on their titles, IDs, and specific content within the document text, and structure the data in a relational/hierarchical repository.
This is equivalent to arranging agent documents in a paperless register with lightning-fast access for future references.
#Derive Target Data
The OCR Engines and Document Parsers can read and capture text from documents and store them in the required format.
A manual process will require reading the document, entering data in a register, and then calculating it. Sounds tedious (even if it’s on excel sheets), isn’t it?
#View Results According to the Selection Criteria
Dashboards allow viewing the output in a decision-ready format.
MaxBupa, a leading health insurer uses an automated solution to process inbound documents for qualifying insurance agents into their distributed-sales network.
Organizations can use some or all of these tools depending on how much they want to automate insurance agents onboarding. Custom workflow automation tools are available for enterprizes, and tailor-made considering the sophistication of the insurance industry.
For customized insurance agent onboarding software solutions, feel free to contact us at hello@mantralabsglobal.com
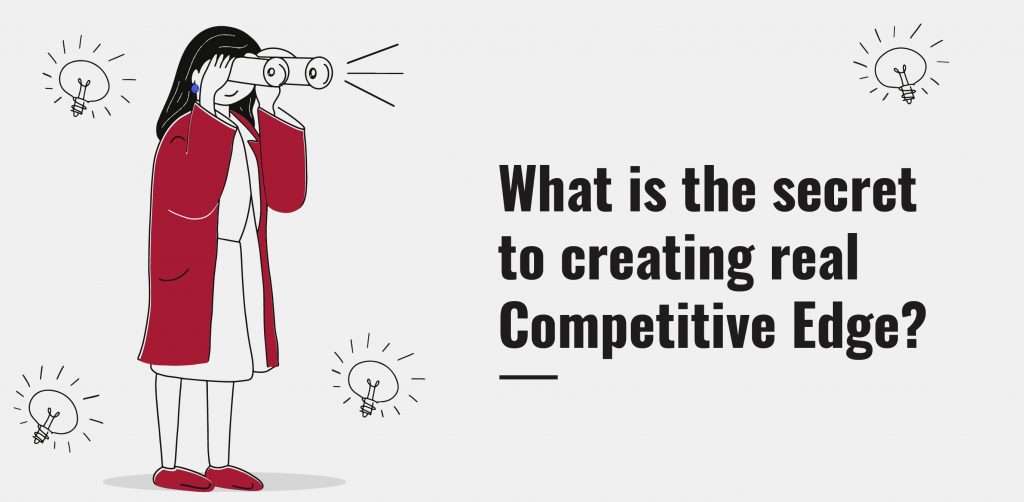
Join our Webinar — AI for Data-driven Insurers: Challenges, Opportunities & the Way Forward hosted by our CEO, Parag Sharma as he addresses Insurance business leaders and decision-makers on April 14, 2020.
Agents (humans) are important too
The traditional model of agent-to-customer communication is not dead. 57% of Indian customers still prefer buying insurance policies through agents. Many a time, agents are the touchpoint between customer portal (technology) and the customer.
From an organization’s perspective, automated systems also ensure effective data management, which gives their agent easy access to customer information and company policies and documents; bridging the knowledge gap.
Why to Automate Insurance Agents Onboarding?
Removing the unnecessary layers of complexity and automating processes can help insurance companies interact with more potential agents and set stricter selection criteria.
Successful onboarding can help establish a strong relationship between agents and insurance companies. According to research from Brandon Hall Group, organizations with an efficient onboarding process can improve new-hire retention by 82%. Also, finding the right person for your organization can improve the agents as well as overall enterprises’ productivity by 70%.
Knowledge thats worth delivered in your inbox