Architecture is perhaps the most complex discipline operating in more dimensions than any other coordinated human activity. However with the advancement of artificial intelligence, like every other profession, architects to are worried about the level of automation that has already taken away specific tasks from their roles.
While the ‘Humans are hooked and Machines are learning’, AI and ML are disrupting all manner of industries. Although AI has taken decades to go from crazy lab demos to a finished consumer product — today, there are immense possibilities for the industry to be augmented and enhanced by artificial intelligence.
The earliest sense of advancement in the construction field came with Building Information Modelling (BIM) — a term that has existed since the 1970s, but came to its penultimate fore in the early 2000s, when Autodesk began popularizing the tag.
The resulting by-product was the BIM software which is a type of intelligent 3D-modelling process used by architecture, engineering, and construction (AEC) practitioners to design and construct any kind of infrastructure. BIM software includes computer-aided design (CAD software) tools and libraries specifically targeted toward architectural design and construction and goes beyond traditional drawings to generate a fully digital model.
Over several years the BIM (Building Information Modelling) software has had a huge influence on the day-to-day operations undertaken in an architectural firm.
The Parametric design or the programming architecture can scrape through several design styles in no time and can come up with a perfect Zaha style building plan — that would otherwise take years to be designed.
Over the last few decades, BIM has transformed the roles of engineers, contractors, architects, developers, and consultants by allowing them to communicate the same language and collaborate better. It has quite literally revolutionized both the design process itself and the designs themselves.
BIM software produces an immense volume of big data, so much so that most architecture firms and their consulting partners don’t know what to do with them. Once AI permeated the technological landscape and bled over into every imaginable business use case — the industry learned to create value by collecting, organizing and storing building-related data (collected from models, simulations, etc.) It is now widely believed, that the scope for innovating the most optimal designs for each construction project becomes completely conceivable.
AI BIM = Optimized [Affinity]
When ‘parametric design’ technology is combined with AI that can actually use 6D BIM-models, and can record the whole life cycle of the building — it can come up with better decisions and insights into project execution by learning from the mistakes of the past.
Today, there are machines that can run through an infinite number of datasets, simulate for each model, pick the best option, verify its efficiency and continue to learn and communicate when introduced with the new autonomous building technology.
AI is the next frontier for architecture
Changes in the demographics, technology and business models have opened up a plethora of far-reaching opportunities for architects to explore areas like urban housing in more ecosystems than ever before.
Let’s have a look at some architectural products augmented and enhanced by AI.
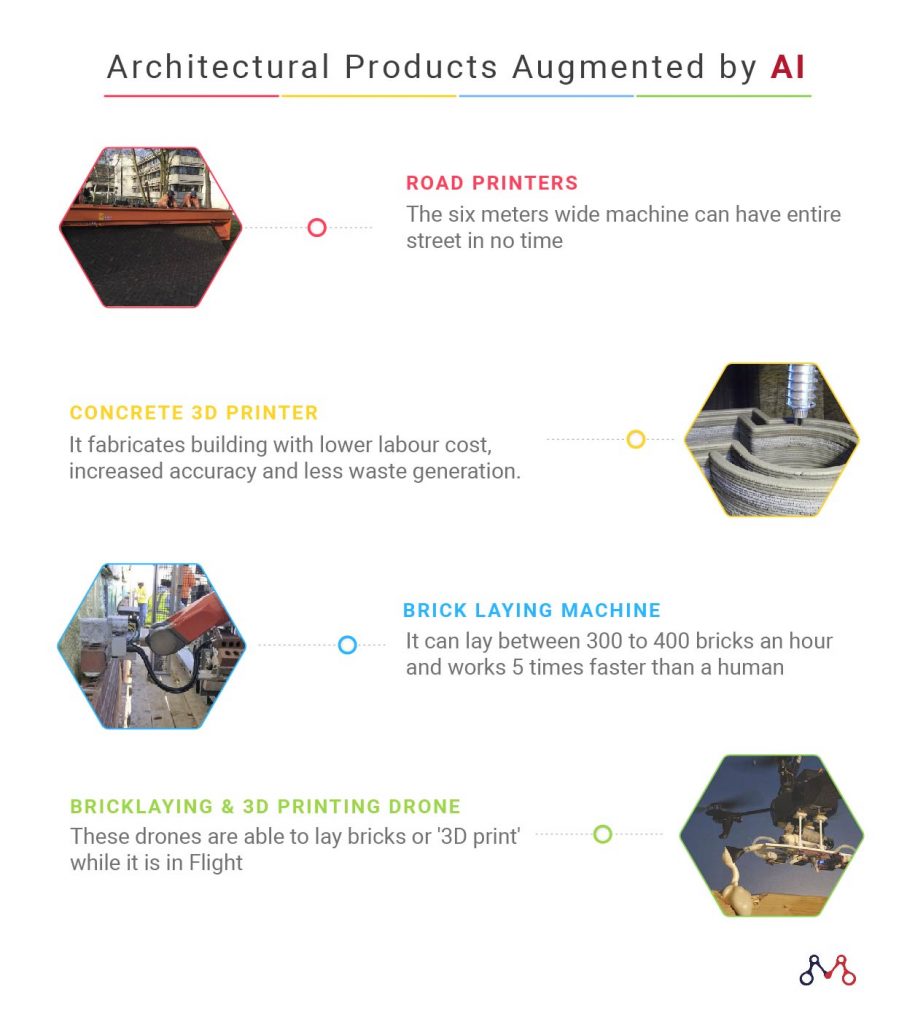
Road Printers
The six meters wide machine that can pave entire streets at once. Naturally, the stones fall on the road directly into the appropriate pattern. The device is simple to handle and can finish the work in no time.
Concrete 3D Printers
3D printing as a core method to fabricate buildings or construction components. At a construction scale, it will have a wide variety of applications within the private, commercial, industrial and public sectors. The concrete 3D printers enable faster construction, lower labor costs, increased accuracy, greater integration of function and less waste produced.
Brick Laying Machine
The bot can lay between 300 to 400 bricks an hour, compared to a human which can only lay around 60 to 75 bricks an hour. It works 5 times faster than a human and can alleviate the labor shortage.
Brick Laying & 3D Printing Concrete Drone
Though in its infancy, researchers from Imperial College London have taken the first step towards making this a reality with their work on a drone that is able to ‘3D print’ while it is in flight.
However efficient bots may be, it will always lag in understanding the personality and the character of the customer — and this is where humans intervene.
Architects with the help of AI can create something different from the one-size-fits-all range of products already in the marketplace, to create more personalized solutions that perfectly align with user needs — but it is the imperfections in our creative decisions that truly makes something personal and truly unique.
What is your opinion about AI in architecture? Do you think AI will either augment or eliminate every profession in the near future?
Let us know by commenting.
To know us in person, reach us on hello@mantralabsglobal.com
Knowledge thats worth delivered in your inbox