The technological advancements brought forth by insurtech will soon become routine for brokerage offices within the next few years. Digital-first approaches have finally trickled down, turning ripe for adoption for this major distribution channel. However, broker adoption has still not caught pace with their agency counterparts.
According to a 2019 report surveying independent insurance brokers across the US, Canada & the UK, the average for digital technology adoption at an independent brokerage is only around 43%, even though nearly 96% of them (almost universally) use a broker management system for indispensable day-to-day operations. Interestingly, over 80% don’t offer any form of ‘mobile apps’ or ‘self-service portals’ for customers or staff.
Today’s insurance customers are younger and prefer digital over traditional channels — leaving a lot of unmet gaps in the value chain. The report also identified key areas where adoption is growing — such as capabilities in workflow process management, document management, sales opportunities & prospect tracking, one system-one view visibility into all departments among others. For example, the downside to not outfitting your broker operation with employee mobility tools alone translates to over 30% reduction in staff productivity.
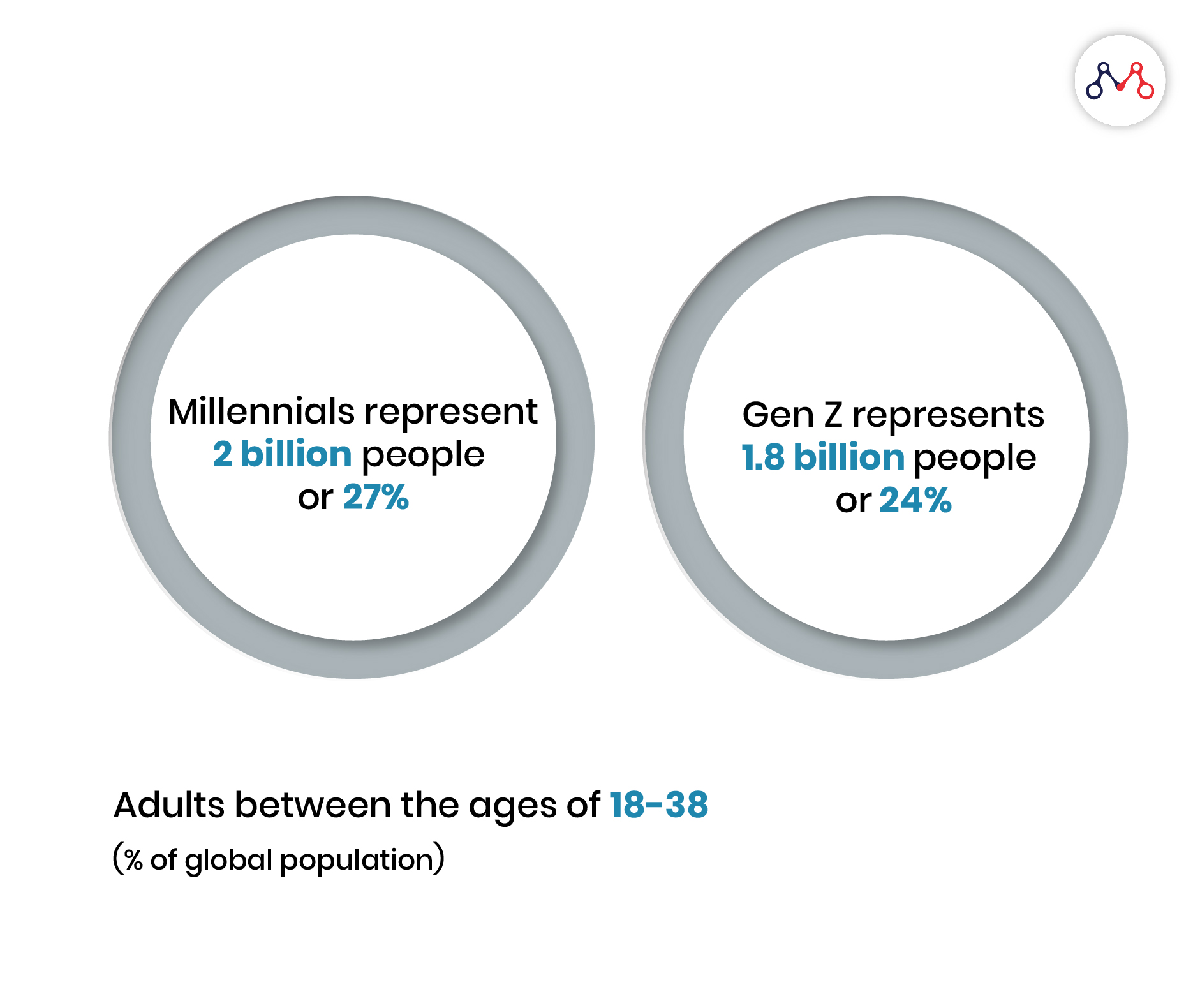
Meanwhile, brokers are facing a whole new set of challenges — Insurance is being built for digital and the audience is changing. Gen Z and Millennials will form the core of their target demographic. A fully online brokerage can benefit these potential customers through simple end-to-end policy administration and by fine-tuning the customer journey.
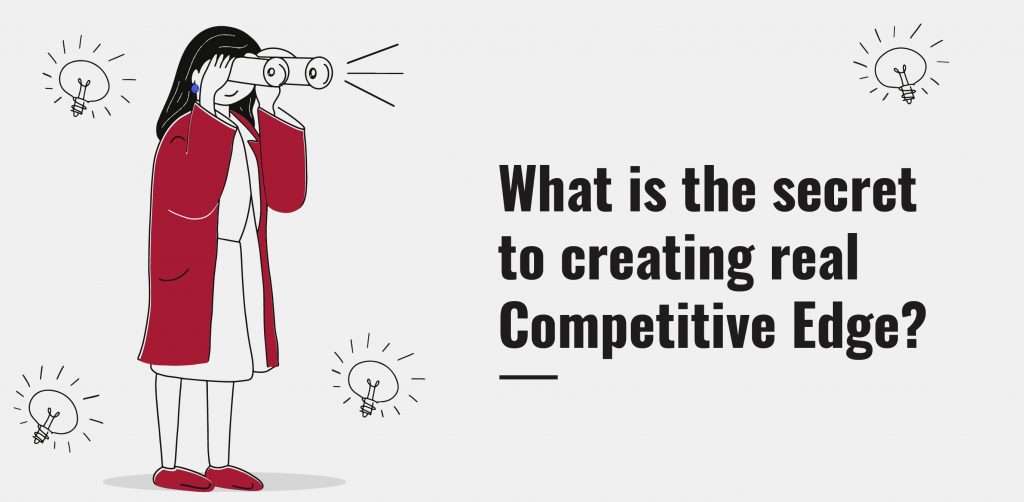
Join our Webinar — AI for Data-driven Insurers: Challenges, Opportunities & the Way Forward hosted by our CEO, Parag Sharma as he addresses Insurance business leaders and decision-makers on April 14, 2020.
While brokers are not involved in the manufacture of insurance products or the evaluation of risk, several other value chain functions are being performed through brokers now — of which managing the customer relationship is pivotal.
There is a lot of data across the lifecycle to look at, which necessitates the need for advanced analytics in order maximize the opportunities to up/cross-sell. At present, data analytics is widely under-utilized among most insurance brokers leaving them blindsided to customer needs.
The Case for a ‘Digital’ Brokerage
A digital broker business is built on these foundational blocks — robust broker management system, seamless mobility tools for employees, insurer connectedness, self-service portals, smart customer apps, advanced data analytics and the cloud.
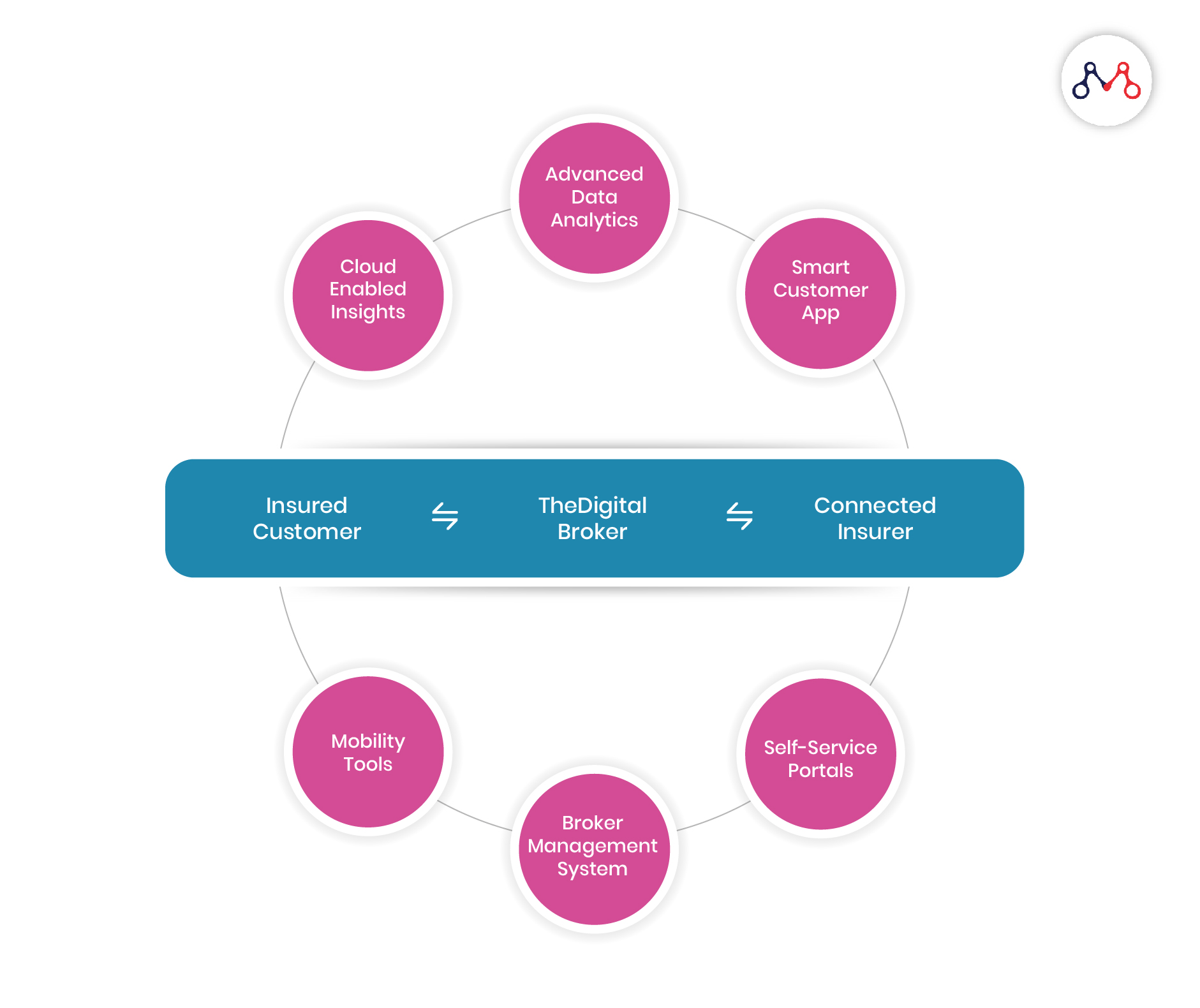
Taking the entire business model online requires the right business advisory and technical roadmap, without which the transformation can leave you with unwarranted gaps in the operating structure. This is where Artificial Intelligence can play a critical role in securing brokerages to be future-ready. The digital broker has to be outfitted with a staunch selection of AI-enabled tools that provide better business visibility, more unified workflows and eliminates time spent managing and updating divergent systems.
Analysing big data (predictive analytics) and social media using AI can offer real-time insights for measuring risk, immediate demands and possible life changes for customers. For brokers, this translates to an enhanced ability to justify value to clients and ultimately retain those customers.
According to a recent EY report on the state of digital brokerages, ‘digital onboarding tools’ and ‘sales leads & application tools’ were identified as attributes with the lowest satisfaction among brokerages. There is a growing sense that these tools need to be a cut above the industry benchmarks — in order to improve the digital relationship with a customer or prospect.
The Digital Broker can also leverage automation to improve efficiency in agent productivity and document handling processes. For instance, enabling employees with remote digital tools empowers them to quickly take action – from quoting prospects to providing policy details and managing claims for existing customers — especially when they need it most.
Brokers, just like insurers and agencies, need next-gen customer engagement solutions in order to maximize real customer lifetime value. Technologies like Artificial Intelligence have the potential to enhance several facets of the business from reducing back-office processing times and intelligent lead allocation to designing better customer facing products. Improvements achieved through the deployment of AI can create significant gains in operational efficiency and RPE (revenue per employee).
To learn how MantaLabs can help your brokerage begin its digital transformation journey, reach out to us on hello@mantralabsglobal.com.
Knowledge thats worth delivered in your inbox