The machines are learning. Slowly, sure, but they are learning and we (humans) are the ones teaching them. We tell the machines how they should learn through the algorithms we write, and then feed them an enormous amount of data, so that it trains endlessly. Data labeling (the process of augmenting unlabelled data with meaningful and informative tags), is a necessary part of machine learning and sadly there’s a simple reason behind the use of a lower-wage workforce to train ML (Machine Learning) models — you only pay them half as much. The market for AI data preparation is projected to leap from $500M in 2018 to $1.2B by 2023.
Data is the only real fodder for any type of AI system. The more it trains on large amounts of ‘good data’, the faster it learns. Behind every piece of machine learning code intended to solve real issues, is a network of digital construction workers bearing the burden of building the foundation for AI — preparing data. For example, AI systems are trained to recognize objects. Data Labelers upload, categorize and cluster millions of images — just about everything from people, animals, buildings, plants, cars, signs, shapes, and things. In doing so, you now have an AI system that can begin to recognize these objects in the real world.
Again, for example, an algorithm meant to classify images of animals uses a large volume of images of different types of animals (dogs, leopards, giraffes, zebras, etc.) to train the model. These images will be labeled and classified for the model to work. A data labeler typically performs this essential function. It annotates the images with the right answers and transforms the dataset into a format suitable for machine/ deep learning.
Data Enrichment for Training ML Models
The real underlying aspect to machine intelligence is ‘the human’ in the AI loop — and it isn’t going away anytime soon either. Functions like data labeling are vital for AI quality control. Big Tech firms readily outsource these tasks to parts of the world where the minimum wage is significantly lower in order to meet extremely ambitious goals within budget. Data preparation and engineering tasks represent over 80% of the time consumed in most AI and machine learning projects.
For instance, small data labeling companies in Kenya (and others spread across Africa) are working with large American & European firms to help them classify and organize millions of datasets. The task involves highlighting and labeling images of vehicles, traffic lights, landmarks, road signs and pedestrians captured by cameras fixed on autonomous vehicles so that these machines can become aware of the objects around them.
Bounding Boxes (tagging images for machine or deep learning models)
Image Segmentation (recognize objects of different shapes, sizes, and positions)
(source: clickworker)
Automation (the precursor to true AI) has put low-skilled jobs at supposed “extinction-level” risk for several decades now, as self-driving cars, rules-based process bots, and speech recognition will continue to exacerbate this trend. In reality, the advances of digital industrialism are not new, neither is the elimination or replacement of low-skill jobs with newer low-skill jobs.
Sebenz.ai, a South African AI firm, is trying to create job opportunities for people throughout Africa leveraging the growing demand locally for data labelers. They have produced a Machine Learning ‘labeling game’ that allows people to earn money on their phones by labeling training data for ML models. Using this innovative approach, Sebenz is able to create labeled-data with real-time responses almost in parallel to train these models accurately.
According to the firm, it takes 10,000 hours of audio to train a speech-to-text model. With 1 data labeler, it would take 65 months, but with 10,000 people it would be ready in a few hours. In return, the data labelers are compensated around $16 per day, (minimum wage in the African continent is only a paltry $3 per day), albeit affording them the opportunity to make a better living. Most of the people drawn to data labeling jobs are often unskilled workers and live below the poverty line.
According to a 2018 KPMG research report, 5% or more of the global workforce will be replaced by automation within the next 2 years.
When Silicon Valley first began importing ‘cleaned’ data in bulk at nearly a fraction of the price, then it would otherwise cost them in their own markets — it wasn’t initially received as the modest competitive advantage as it is today. However, looking ahead at the ‘future of work’ and the role of Big Tech in shaping the informal economy — the low skilled jobs fueling automation and AI will soon become automated themselves, creating newer jobs and roles for people en masse to move into, yet again.
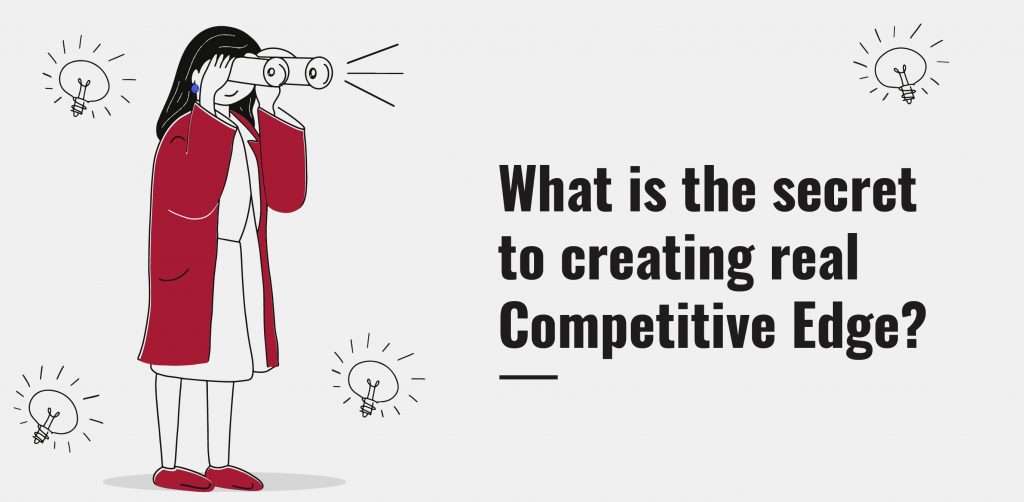
Join our Webinar — AI for Data-driven Insurers: Challenges, Opportunities & the Way Forward hosted by our CEO, Parag Sharma as he addresses Insurance business leaders and decision-makers on April 14, 2020.
AI is shaping the future of enterprises and consumer-services in affordable and scalable ways. To learn more about how we can transform your AI journey, reach out to us at hello@mantralabsglobal.com.
Knowledge thats worth delivered in your inbox