Human Behavior is inherently hard to predict and mostly irrational. Infact, this irrationality is often overlooked because it offers no meaningful insight or patterns behind our motivations.
In the early 70’s, Israeli-American economist Daniel Kahneman challenged the assumption that humans behave rationally when making financial choices. His research explored the fundamentals of how people handle risk and display bias in economic decision-making. He would later be awarded the Nobel Prize for his pioneering work which provided the basis for an entirely new field of study called Behavioral Economics.
Standard Economics assumes humans behave rationally, whereas Behavioral economics factors in human irrationality in the buying process.
Along with another scientific approach to studying natural human behaviors (Behavioral Science), both these fields became particularly useful to the financial industry early on. By understanding the deep seated motivations behind people’s choices, a specific interaction can be designed to influence an individual’s behavior — also known as behavioral intervention.
By finding meaningful patterns in Big Data, usually performed by a data scientist, businesses are able to leverage analytics and behavioral customer psychology. The outcomes of these insights can help business owners learn about the customer’s true feeling, explore behavioral pricing strategies, design new experiences and retain more loyal buyers. This is why Behavioral Scientists have become highly sought after over the last decade.
The Rise of the Behavioral Scientist
Take for instance Dan Ariely, who is a Professor of Psychology & Behavioral Economics at Duke University, and also serves as the Chief Behavioral Officer of Lemonade — the World’s biggest Insurtech. Ariely observes that human behavior is ‘predictably irrational’ and constantly exhibits ‘self-defeating’ characteristics. There is a lot of value in studying these behaviors, for many organizations, to encourage positive ones, dissuade dishonesty and improve the underlying relationship.
The ‘dissuading dishonesty’ part is particularly useful for Insurance carriers. For a business that fundamentally deals with both people and risk, Insurance is endlessly plagued by fraud. Insurance fraud losses were estimated around $80B in 2019 alone. On the other hand, legitimate claim instances can at times be overlooked due to the lack of evidence or nuances in the finer policy details.
To combat fraud during the claims process, Ariely added a simple ‘honesty pledge’ agreement before the beginning of the claims intimation process. A customer signs the digital pledge, and is then asked to record a short video explaining the incident for which they are requesting the claim.
The process seems naive but it’s backed by tons of data and science — a byproduct of decades of research work put into psychology and behavioral economics.
So, How are claims being driven by data science?
How do insurers capture honesty from their customers?
The answer is priming.
By enforcing an honesty pledge, Lemonade was able to bring down the likelihood of fraudulent claims being intimated for. In other words, they made it harder for customers to lie. The hypothesis that works is: Don’t blame people for mistakes in decision making, it’s on the designers of the system.
After the customer got done with their video recording, Lemonade ran 18 anti-fraud algorithms against the claim to check its veracity and a payment was made in a few seconds.
Behavioral Priming in Insurance
Behavioral work is built on strong academic research that identifies aspects that influence the buying process. ‘Nudges’ are a perfect example of behavioral priming at work. Nudge theory (a concept within Behavioral Science) identifies positive reinforcement techniques as ways to influence a person’s behavior and ultimately their decision-making.
For example, according to a study published in the Journal of Marketing Research, research subjects who were shown an aged image of their faces allocated twice the amount to their retirement savings when compared to people who were shown images of their current younger selves.
In this case, the ‘nudging’ technique was effective in driving retirement planning behavior among the test group.
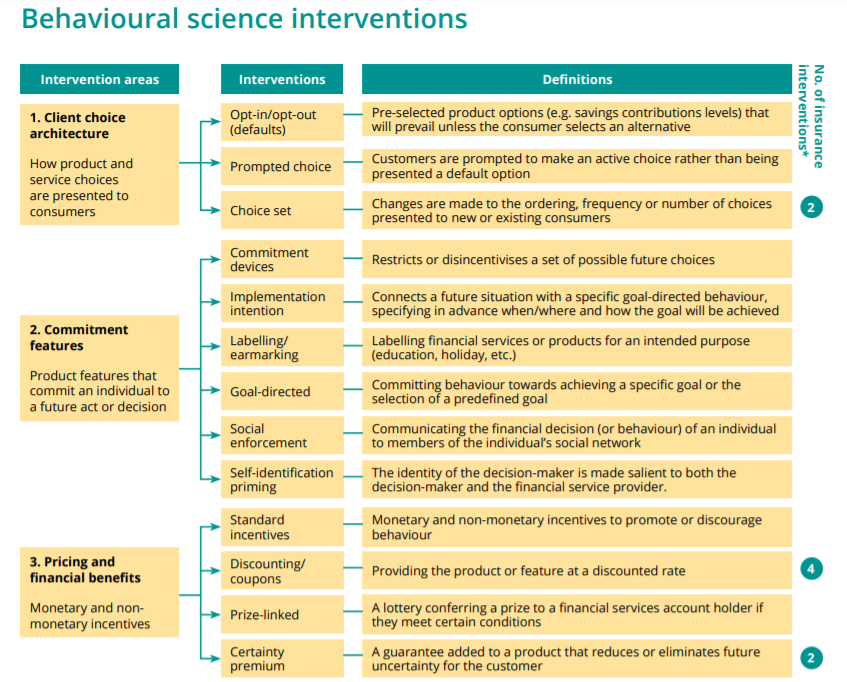
Source: Centre for Financial Inclusion
Behavioral Economics also stipulates that once you start doing something, you are more likely to continue doing so. This is how Netflix uses subtle nudges on their platform, where after each episode a prompt asks if you would like to continue watching the show.
Deriving New Value
Swiss Re’s Behavioural Research Unit outlines five promising areas where behavioral economics can create new value for insurers.
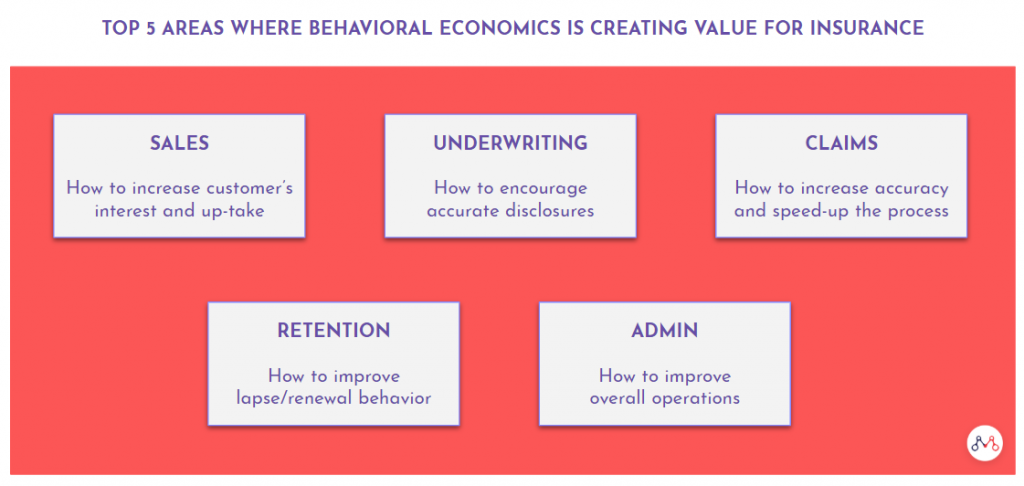
Digital businesses are gradually realizing the limitations of human and machine systems without any real intelligence or computing power behind it. Between human prone errors and the scalability challenges of traditional technologies, a new mechanism is required to learn and adapt better.
Behavioral Science interventions in insurance can help carriers align their strategies with the true needs of their customers. Using the insights posited from advanced machine learning models, the right behavioral intervention can bring about changes to real-world insurance demand behavior that closely matches the benchmark model.
Also read – how InsurTech beyond 2020 will be different?
Knowledge thats worth delivered in your inbox