Malaysia, drawn by its strong economic growth, expanding middle-class income and rising insurance penetration levels, is witnessing a new era of innovation – with AI leading the charge in bringing new and intelligent technologies to the mass-market.
According to Bank Negara, the country’s regulator of banks and insurers, life insurance penetration rate stood at 56% in 2018. Foreign insurers have been highly keen in this market despite lingering regulatory uncertainty over the sector’s foreign ownership rules, currently set at a 70% cap.
While ‘motor’ remains the largest class of insurance with a market share of 45.6%, followed by fire at 19.2% and marine, aviation and transit (MAT) at 8.2%; Takaful has been outpacing conventional insurance in the Islamic peninsula.
(Takaful refers to Islamic insurance products.)
Islamic insurance penetration rate in the country will likely touch 16% in 2019. In financial dealings, ‘takaful’ firms follow religious guidelines including bans on interest and monetary speculation and a prohibition on investing in industries such as alcohol and gambling.
Growth in the takaful business in Malaysia, the world’s second largest Islamic insurance market after Saudi Arabia, is backed by government efforts to reach out to the general consumer with affordable insurance coverage and the potential use of better technology as a disruptor.
AI is already poised to play a crucial role in Malaysia’s next big step. By 2021, Artificial Intelligence will allow the rate of innovation to almost double (1.8x) and increase employee productivity improvements by 60% in Malaysia, according to an AI study put forth by Microsoft & IDC-ASEAN Research Group.
While seven in 10 business leaders polled agreed that AI was instrumental for their organisation’s competitiveness, only 26% have embarked on their AI journeys. Those that have adopted AI expect it to increase their competitiveness by 2.2 times in 2021. Though, everyone agrees – every single interaction from here on is going to be digital.
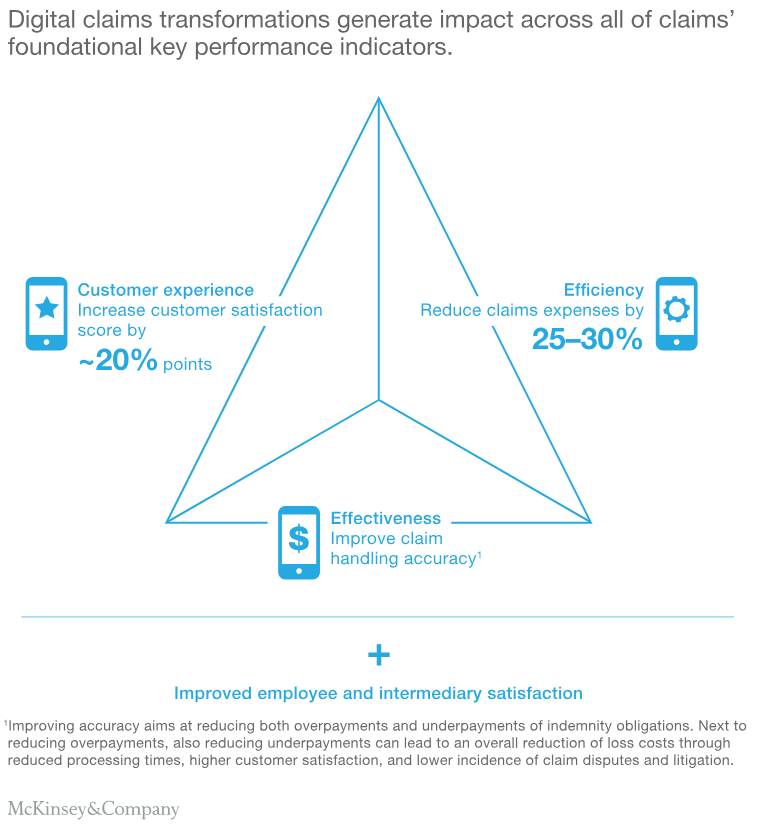
Malaysia is also moving towards a cashless society with infrastructure being put into place to facilitate e-payments which have more than doubled per capita from 2011 to 2019. For this, banking solutions in the region have ramped up digital investments so customers can take advantage of convenient and secure banking.
Intelligent Claims Automation
For insurers, claims settlement represents a large customer service touch point. However, taking a customer seamlessly through the claims resolution process is not always going to be simple.
Being an AI-driven insurtech enterprise means being able to fully utilize data and optimize business processes with powerful algorithms, creating the space for data-driven decision making. With AI, the claims process can be augmented using chatbots to convey support and status of a claim, and Machine Learning (ML) that can study large-volume patterns to reveal insights and detect fraud. Claims automation can be achieved at part or whole of the settlement process.
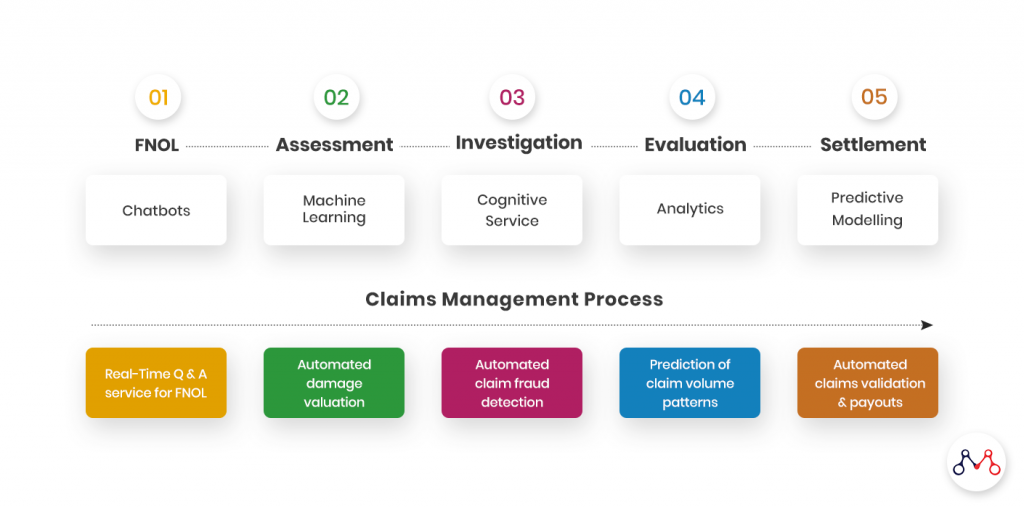
The Malaysian Insurance market is already witnessed to big insurers rolling out innovative products for customers, such as “Ask Sara” – AIA’s AI-powered enquiry channel that provides instant, real-time answers to agents anytime via Facebook Messenger. Integrating sensors into the value chain has also provided greater rewards with predictive modelling and data analytics, like Katsana – a telematics company that is enabling insurers to provide usage-based insurance based on driver’s performance data. These measures allow for safer, accurate and more affordable risk-based pricing for consumers.
The attitudes of the insurers and younger generations are shifting alongside their Asian peers, to a seemingly more AI-involved future. While the general insurance trade has witnessed nearly stagnant growth over the past several years, AI can help lower overheads and variable costs that will enable insurers to roll out affordable coverage, including to the under-served segment.
Enterprises benefit from our AI-first thinking.
We build AI roadmaps from scratch, guiding you all the way through your next transformational journey.
To learn how, drop us a line here: hello@mantralabsglobal.com
International Insurance Landscape
Knowledge thats worth delivered in your inbox