A new report from Juniper Research has found that annual cost savings derived from the adoption of chatbots in healthcare will reach $3.6 billion (€3.04 billion) globally by 2022, up from an estimated $2.8 million (€2.36 million) in 2017. This growth will average 320% per annum, as AI (artificial intelligence) powered chatbots will drive improved customer experiences for patients.- IoTnow
Experts believe that medical chatbots will play an important role in the healthcare industry soon. While the world is facing the problem of information overload, bots can help in segregating good and bad/right and wrong data. Healthcare chatbots solve the most pressing medicine industry problems-
- Accessibility: The human workforce might not be available in every vital situation. But bots are. Thus making appointments and scheduling simple.
- SOS: Healthcare chatbots can also act as an alarm in the instances of life-threatening symptoms as described by the patients.
- Customization: Bots with NLP capabilities can understand voice and text-based queries efficiently. This is especially helpful for people with visual or speech impairments.
- Integrations: Chatbots are compatible with IoT devices like Google Home, Alexa, etc.
- Personalization: Patient-specific bots can place a call, advise first-aid, and even send notifications/messages to physicians.
Before we delve deep into medical chatbots, let’s quickly look at what exactly is a chatbot.
Chatbots Brief History
The first-ever computer program that could communicate with humans was Eliza, developed by MIT in 1966. Subsequently, with programs passing Turing tests, e-commerce, messaging, healthcare, and other enterprises indicated a deep interest in using chatbots.
A chatbot is an artificial intelligence program that can interact, respond, advise, assist, and converse with humans. It can mimic a two-way communication between two individuals. In the initial phases of chatbot implementation, tasks like — scheduling an appointment and answering fundamental queries, were accomplished. Today, the scope of chatbots is much broader with recommendations, references, diagnosis, and even preliminary treatments.
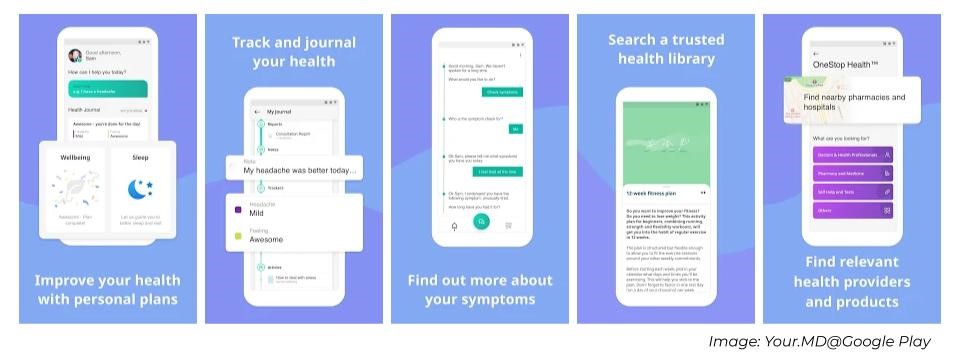
How are Medical Chatbots Reshaping the Healthcare Industry?
Chatbots can help in improving Patients’ engagement and experiences with the hospital/physician. The following are the proven benefits of healthcare chatbots.
Accessible Anytime and Anywhere
Today, it is possible to embed chatbots on websites, mobile apps, and even third-party apps like Facebook and WhatsApp. There’s no need for downloading them explicitly and registration/activation. For instance, Religare, a leading health insurer has its chatbot integrated into WhatsApp.
Generally, hospitals and other healthcare organizations provide chatbot in-built in their app. Apart from usual support, it also helps to secure your medical records in one place. This record can also be shared with the doctor whenever it is required.
There are mental health and therapy chatbots available that provide continuous support to patients with mental illness, depression, or sleeplessness. Wysa — a mental therapy chatbot is one such example.
Chatbots for SOS (Emergency Alarms)
During the instances of life-threatening events/symptoms, chatbots can help in raising alarm automatically. For example, if a person who complains about chest pain does not respond to messages within a stipulated time, an emergency call made by the bot to healthcare/family/friends might help in attending the needful.
Customization
Organizations can customize chatbots to decipher different languages, voice accents, and text patterns. With different modes of conversations, chatbots can simplify communications. For instance, for a person with a visual disability, computer vision could be altered. For people with speech problems, chatbots with NLP capabilities can be beneficial.
Another remarkable development in chatbots that we’re going to witness soon is that of Emotion AI. Soon the bots would be able to understand the user’s emotions based on text, voice, or sentence structure. This feature will be a great help for understanding the sentiments of people suffering from depression or any other kind of mental illness.
Integration with Different Platforms and Personalization
Because of their omnichannel nature, one can easily integrate bots with the web, mobile, or third-party apps, and APIs. They can even work with voice assistants like Alexa and Google Home. With seamless integration, a bot can place a call, advise you on first aid, analyze your medical history and send notifications to your doctor.
Voice-enabled chatbots and multilingual chatbots are disrupting the way customers engage with chatbots. Voice-enabled chatbots increase accessibility and speed up the query process, as the user does not have to type. More than 70% of Indians face challenges while using English keyboard, and approximately 60% of them find the language to be the key barrier in adopting digital tools. Vernacular language support can personalize the services for native users and make the whole process of maintaining medical records a lot easier. For instance, to automate help desk tasks and respond to customer queries, voice-driven chatbots can be integrated.
Indian chatbots like Hitee (designed for Indian SMEs) support several Indian regional languages including Hindi, Tamil, Bengali, Telugu, Gujarati, Kannada and Malayalam.
Video conferencing chatbots can be used by private clinics and healthcare practitioners to converse with their patients.
5 Popular AI Healthcare Chatbots
- mfine: It is a digital health platform for on-demand healthcare services. It offers online consultation (text, audio, video), medicine delivery, follow-ups, and patient record management services.
- Wysa: Developed by Touchkin, Wysa is AI-powered stress, depression, and anxiety therapy chatbot. It helps people practice CBT (Cognitive Behavioral Therapy) and DBT (Dialectical behavior therapy) techniques to build resilience. For additional support, it connects people with real human coaches.
- Mediktor: It is an accurate AI-based symptoms checker with great NLP (Natural Language Processing) capabilities.
- ZINI: It provides every user with a Unique Global Health ID that can be used for managing one’s healthcare information all over the world. It also creates an emergency medical profile for the patient for urgent medical requirements.
- Your.MD: It provides personalized information, guidance, and healthcare support. With an in-built symptom checker, vast medical database, health plans, and journals, it is a certified application for digital healthcare support.
To know more about how AI is innovating the healthcare industry in bringing the digital health era, check out our webinar on ‘Digital Health Beyond COVID-19: Bringing the Hospital to the Customer’ on our YouTube channel.
We specialize in building custom AI-powered chatbots specific to your business requirements. Feel free to drop us a word at hello@mantralabsglobal.com to know more.
Related articles-
Knowledge thats worth delivered in your inbox