The COVID-19 has proven to be havoc in this tech-savvy world. The community of Healthcare and Development has become the epicentre of the World’s attention for the motives of fighting against the disease; providing social services in this pandemic situation and promoting humanity and livelihood above all.
However, on the flip side of the coin, we are witnessing challenges like never before. With the outbreak of this catastrophic pandemic, medical accessibility and safety have become our primary concern, bringing about a paradigm change in the state of the healthcare industry throughout the world.
As goes the old adage, “Necessity is the Mother of Invention”; the healthcare sector, post COVID-19 pandemic; is about to undergo metamorphosis with a plethora of new ideas. Getting accustomed to the lockdown phase, people are more and more acquainted with the use of technology. From 8 to 80 almost everyone has resorted to the digital platform and shall continue to retain the habit post-pandemic. Like other brick and mortar bodies, a huge part of healthcare shall have to move online, too.
AI-powered customer support
The idea of telecommunication in the field of healthcare will see a sudden spike in usage. The number of telehealth consults has risen exponentially during this pandemic and it will multiply manifolds post COVID-19. During this outbreak, with an increase in queries and lack of live agents, AI-powered customer support can be used as the first line of communication. Unlike old IVR’s, AI-enabled customer support shall understand the patient’s needs and converse with them as a live agent.
Vozy’s Lili, is a conversational AI platform for healthcare organizations that alleviates pressure caused due to high call volume. Apart from providing customer assistance, it maintains a complete patient flow and helps monitor the health conditions post-treatment.
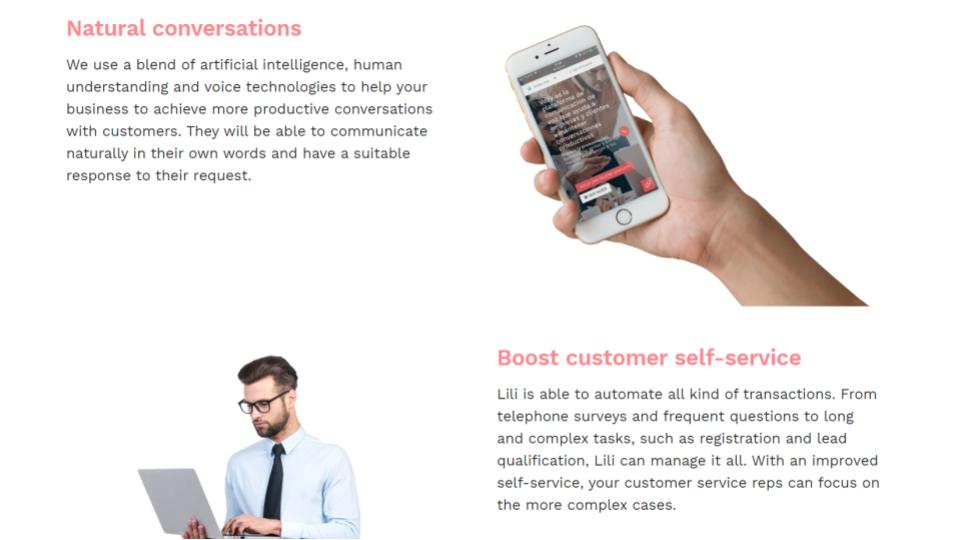
Healthcare professionals are also opting for chatbots for checking symptoms to access symptoms, understand the conditions and accordingly suggest remedies or schedule appointments.
Automation for contactless patient management
While we pull up our socks for a strategic battle, we can promote our major workforce and healthcare by optimizing and digitizing it, sans promoting widespread of this contagious phenomenon.
Data management of patient’s documents not only consumes a lot of bandwidth of medical staff but might also increase the phobia of the spread of coronavirus through touch, post-pandemic.
“End-user organizations adopt RPA technology as a quick and easy fix to automate manual tasks,” said Cathy Tornbohm, vice president at Gartner.
Healthcare applications, like Practo, can not only automate healthcare data management but also provide expert suggested healthcare tips. It connects with the nearest doctors and helps you choose on the basis of feedback, fees and doctor’s profile. It provides affordable healthcare packages, free healthcare tips and many more.
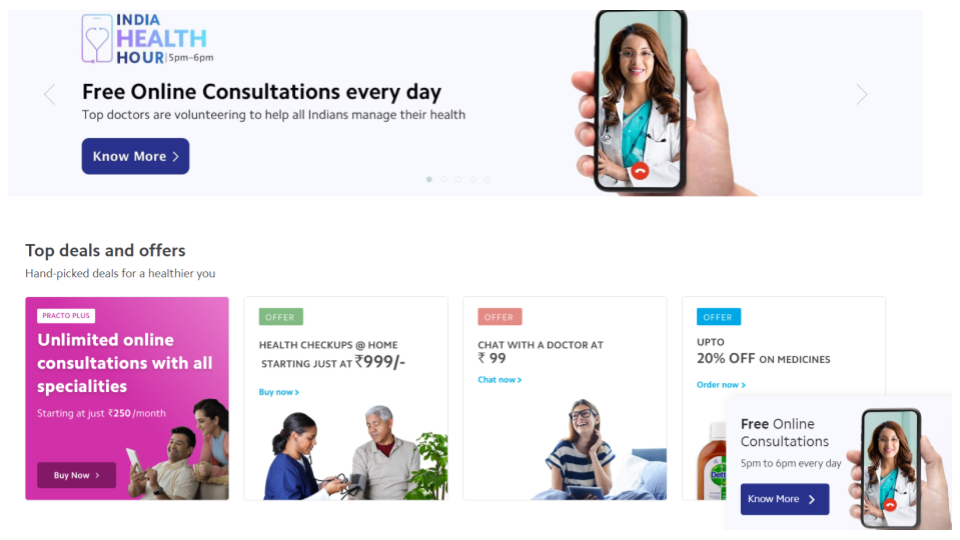
With the implementation of automation in healthcare, it will not only reduce redundancy time but also provide an unbiased and transparent workflow.
[Also read – Are wellness and diagnostic apps transforming ‘Patient Experience’]
Remote monitoring
AI in healthcare is going to be the next big revolution. Preserving human life by implementing robotic operations would be the next big step in the medicine industry. Basic hygiene will become the most important factor and the scarcity of equipment which we are facing will alarm us to prepare in an exponential and not in a linear way.
In radiology, medical professionals examine medical images such as an X-Ray, ECG or a radiogram to diagnose the illness and suggest a solution. With telemedicine being very popular in present times, workstations can be created where radiologists worldwide can consult each other. With the help of AI and machine learning, solutions can be suggested to the medical practitioner.
Neucleus.io is one such web-based work station that provides access to medical images with diagnostic workstation performance.
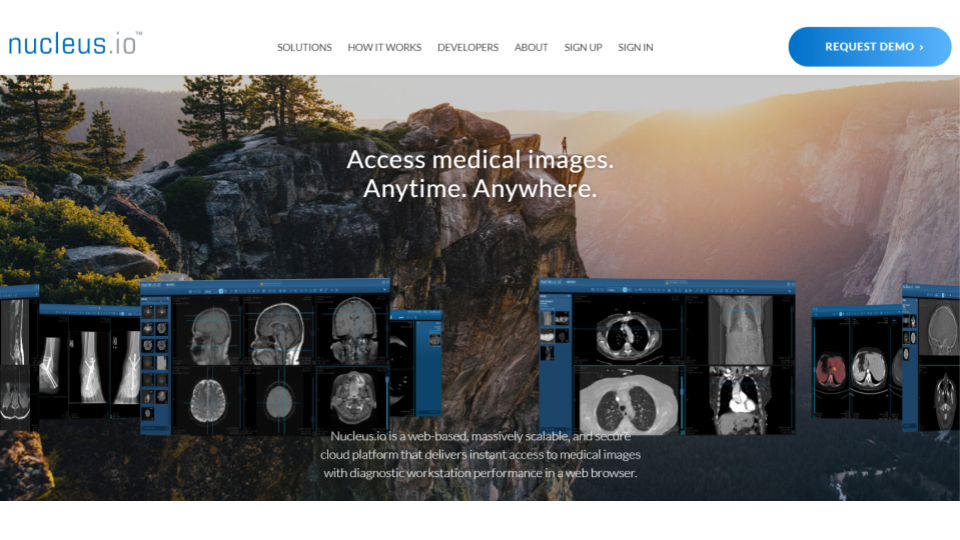
Training neural networks with the results of past attempts can rule out the need to test every combination in drug creation. It can also guide the treatment discovery process and help in telemedicine through drug selection.
To maintain social distancing and contactless patient monitoring, Robot doctors of Canada are already performing real-time ultrasound and helping doctors treat patients remotely.
A different future for the healthcare industry
Post pandemic, more of the typical traditional process requiring human functioning will be replaced by machines, to work more swiftly, providing better results. Thermal sensors will be incorporated in our everyday use gadgets like Mobile phones to allow a thermal scanning process so as to differentiate between normal and ill people on the basis of parameters like body temperature, sweat, facial symptoms, etc.
Digital transformation will be prevalent everywhere post this catastrophe and machines, technologies and AI will become the tools in reshaping the structure of the healthcare industry. If such a situation knocks our door again, we will be all set to sail through the storm.
Check out the webinar on ‘Digital Health Beyond COVID-19: Bringing the Hospital to the Customer’ on our YouTube channel
Knowledge thats worth delivered in your inbox